Kosslyn's Cognitive Architecture
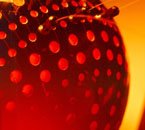
1) Low-level vision - Defined as the component that is driven by sensory input to detect lines, shapes, colors, textures, and depth, this component is topographically organized.
2) High-level vision - Defined as the component that implements mental imagery and object identification, this component is often not topographically organized.
2.A) Visual Buffer - This subsystem of high-level vision explicitly represents edges, depth, and orientation at multiple scales. As the first component of high-level vision, the visual buffer receives its input from low-level vision.
2.B) Attentional Window - This is a "window" onto the buffer, of fixed and presumably less size than the buffer itself, which can adjust its focus on the buffer in three dimensions. It is also subject to a scope/resolution tradeoff in which attending to a larger visual area results in decreased resolution. Window size is changed linearly through either bottom-up, preattentive mechanisms (on the basis of simple physical features) or through top-down attentional shifting. This sends spatial information to the dorsal stream, and object/identity related information to the ventral stream.
2.B.1) Dorsal Stream - this pathway receives information relevant to spatial properties from the attentional window and magnocellular input, and consists of two main stages: spatiotopic mapping and relation encoding.
2.B.1.a) Spatiotopic mapping - this process transforms retinotopic input from the dorsal stream into a spatiotopic mapping: a unified representation of both the size and the location of both objects and their constituent parts. Multiple levels of scale and resolution can also be represented, and outputs are sent both to long-term associative memory and to the encoding processes, as described below.
2.B.1.b) Encoding Process - Receiving input from spatiotopic mapping, this process consists of two stages:
2.B.1.b.i) Categorical relation encoding - based on input from spatiotopic mapping, this left-hemispheric process encodes categorical relations between objects and object parts. These relations are of the kind "above," "next to," "behind," etc. Orientation and size are intrinsically encoded by these relations and their associated nonspecific values: "how far above" one part is from another part can tell you the relative size of that object.
2.B.1.b.ii) Coordinate relation encoding - based on input from spatiotopic mapping, this right-lateralized process represents the specific coordinate locations of objects (or an object's parts) and the specific distances between them. Either global or local coordinate systems can be used; for global coordinates, there's only a single point of origin, whereas in local coordinates every object can be represented in relation to another object (in a sense, every object is a point of origin). This process is heavily used in navigation.
2.B.2) Ventral Stream - this pathway receives information relevant to an object's physical characteristics from the attentional window and parvocellular input, and consists of three primary stages:
2.B.2.a) Preprocessing - this step extracts invariant properties from ventral input, including parallelism, geometric properties of edges and corners, etc. Bayesian methods can determine whether these properties occured by chance alone; those that are considered "nonaccidental" (aka "trigger") features are then combined into invariant perceptions of shape, which is matched against stored representations of shape below.
2.B.2.b) Pattern Activation - Information from Preprocessing is matched against modality-specific representations of previously-seen objects. This system must be capable of both generalization and identifying unique instances of objects. It then sends the name of the potential object, along with a confidence rating, to long term associative memory, with which it engages in a kind of dialogue. The sizes, locations and orientations of both stored and the input representations can be changed until the best match is found.
2.B.2.c) Feature Detection - this system works primarily with color (though probably also texture and intensity) to extract features unrelated to shape. Those detected features are then sent directly to associative memory. This subsystem is admittedly coarse.
3) Long-term associative memory - this poly-modal, non-topographic component receives inputs from a variety of dorsal and ventral subsystems, including Spatiotopic Mapping, Relation Encoding, Pattern Activation, and Feature Detection. All the various pieces of information corresponding to an object become associated with one another through a parallel process of constraint-satisfaction, where both object properties and spatial properties are integrated into a propositional representation of object identity. This information feeds back into the pattern activation subsystem, where visual memories are stored.
4) Hypothesis testing system - this component violates the hierarchical decomposition principle of Kosslyn et al., because it is not just one area but consists of an interaction between many areas. It consists of two winner-take-all competing subsystems operating in parallel, and a third final attention-shifting process:
4.A) Coordinate property look-up - this subsystem returns the spatiotopic coordinates of the parts of a specific object, based on representations in long-term associative memory. This information is then sent not only to the attention-shifting subsystem (below) but also to the pattern activation subsystem, in order to bias perception in favor of a hypothesized object. It is this latter pathway that accomplishes object recognition through a process of constraint-satisfaction between perception and prediction.
4.B) Categorical property look-up - this subsystem provides local coordinates for the parts of a specific object that has been "looked-up" in long term associative memory. Outputs from this process are sent to the pattern activation subsystem, in order to bias perception in favor of a hypothesized object. Outputs are also sent to the categorical-coordinate conversion subsystem, below.
4.B.1) Categorical-coordinate conversion subsystem - receiving input from categorical property look-up, this system transforms object size, taper, and orientation information into a set of specific coordinates. It does so through a two-stage process:
4.B.1.a) Open-Loop process - this outputs a range of coordinates to the closed-loop process via a "fast and loose" type algorithm
4.B.1.b) Closed-Loop process - this "fine tuning" process zeros in on specific coordinates to which attention can be shifted, based on location closest to current position of attention window.
4.C) Attention-shifting system - receiving input from the look-up systems above, this process shifts the attentional window, and moves the head, eyes, and body as appropriate to the new coordinates for attention. Since the attentional window is retinotopic, this system must convert coordinates from spatio- to retinotopic and then send the appropriate information to other regions. This is accomplished in two phases, and three very coarse subsystems:
4.C.1) Phases
4.C.1.a) transform coordinates from spatiotopy to retinotopy
4.C.1.b) fine-tune the new attentional window location based on attentional feedback
4.C.2) Subsystems
4.C.2.a) Shift attention to position in space - accomplished by the superior colliculus
4.C.2.b) Engage attention at that position - accomplished by the thalamas
4.C.2.c) Disengage attention as appropriate - accomplished by parietal lobe
3 Comments:
Hi Chris,
Just to let you know, I've been enjoying reading your blog a lot, it is in fact one of the best sources of information I found about research in intelligence; and always a very interesting read. I'm generally not leaving comments as I don't have much insight yet about the topics you discuss, but I wanted to thank very much for making this resource available.
Keep up the good work!
-- Christophe
Wow, what a compliment!
But I have to tell you, I am really just learning about alot of this stuff myself: it's entirely possible that my hypotheses are outlandish (well, that's actually pretty likely) and/or my speculations are completely wrong.
So I hope no one's hesitating to offer facts (or anecdotes, or even ungrounded opinions!) that bear on my hypotheses & speculations...
Thanks again christophe.
-Chris
Hi Chris,
I liked your blog too. I am just beginning to persue the study of human visual system, most importantly how humans understand an image. I am thankful to you for sharing your insights.
Post a Comment
<< Home