From Inhibitory to Excitatory and Back Again
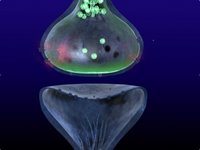
Most neuroscience texts will tell you that a neuron is either excitatory or inhibitory; that is, it will release either an inhibitory neurotransmitter (such as GABA) or an exictatory one (such as glutamate). Yet Erik Fransen has observed co-release of both excitatory and inhibitory neurotransmitters from the same synaptic terminal. He has also observed "backpropagating dendritic action potentials" which can actually cause neurotransmitters to flow backwards from dendrite to axon! This then causes "conditioning depression" in which the presynaptic neuron can go below 50% of its initial amplitude.
So, what happens for the really freakish neurons that undergo both co-release of transmitters AND show conditioning depression? From evidence with computational models, neurons with both features can actually switch between the release of GABA and glutamate. This reversal can even be stable over time - presumably until the next backpropagating dendritic potential.
How do these changes affect neurocomputation? It's difficult to say. The backpropagation algorithm has been a feature of artificial neural network models for quite some time, even though it has been criticized for a lack of biologically plausibility. Co-release, on the other hand, is certainly not a standard feature of any artificial neural network; it was thought to be impossible. Given the emergent nature of neural processing, and the enormous number of neural interactions that could be affected, it's difficult to speculate on how these observations may affect neural computation.
It is far easier to speculate on the implications for modeling. Obviously, biologically plausible models will need to be updated with this surprising new feature of neural networks. Secondly, the philosophy of "biological plausibility" may require rexamination. As a form of "Occam's razor," it can be useful in constraining neural network models, but is still risky in that we may intentionally underestimate the features of neural networks.
Further, plausibility is a difficult thing to judge when we're still guessing about so much of neural computation! There may even be some validity to the idea that Occam's razor shouldn't apply to complex biological systems, given that adaptation generally requires complication rather than simplification to existing biological structures. And how can we even posit the "simplest" explanation for a phenomenon, like intelligence or consciousness, when we not sure of any mechanism that would cause these to occur in the first place?
0 Comments:
Post a Comment
<< Home