An Informal Integration of Object Recognition Models
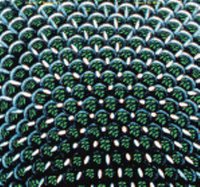
Any complete model of pattern recognition must account for viewpoint-dependent reaction times in object recognition, and yet be able to simulate viewpoint-independence as exposure to a given object increases. Many early theories of object recognition stressed the view-invariant aspect to object recognition, citing the impressive human ability to recognize a new instance of an old object despite the kinds of changes in orientation, size, and lighting that occur in everyday life (Biederman, 1995). Other data showed that naming times for basic-level categories is invariant across changes in viewpoint, suggesting that the primary mechanism of object recognition is view-independent (Biederman & Gerhardstein, 1993). Upon closer inspection, however, human object recognition is not perfectly robust to pattern variability.
For example, subjects can more quickly recognize objects from specific characteristic views than other less characteristic views (for example, a cow may be easier to recognize from the side than from the bottom). Although some adaptations of view-invariant approaches can account for this data, experiments such as those conducted by Tarr (1995) are much more definitive. Tarr clearly showed that the time required to recognize a novel view of some object is linearly dependent on its angular displacement from the closest previously studied view. In other words, even after extensive experience with an object, one can only recognize it from a new perspective by mentally transforming it to match a memorized view.
The nature of this transformation relies critically on the kinds of information available to it. In the first stage of my proposed model, image preprocessing extracts surface-based primitives in parallel from basic color, texture, orientation, and contour information available in earlier visual areas (similar to Wolfe & Horowitz’s 2004 treatment of guiding attributes). This proposal is compatible with behavioral evidence from IT-lesioned monkeys; though unable to recognize objects in most tasks, they were able to partially succeed in some object recognition tasks by differentiating between objects on the basis of contour (Farah, 2000). This evidence suggests that contour-based information is available prior to IT, and therefore that object-recognition processes rely on representations that are extracted after contour. This notion is also compatible with Kosslyn et al’s (1990) “preprocessing” stage in the ventral pathway.
In the second stage of this model, the incoming surface information is transformed to familiar or characteristic views. This transformation can take the form of mental rotation, view interpolation, or linear combinations of surfaces (Tarr & Bülthoff, 1998). This normalization step allows for incoming visual data to be matched against multiple views of an object, such that if one has enough visual experience with an object, it might be recognized from most novel angles with nearly equal ease. Object transformation is terminated when the surface-based information (in the form of graded activation) sufficiently matches a familiar view (in the form of connection weight patterns) driven by prior experience; in cases where the incoming information is already close to a familiar view, no such transformation is necessary because activations will match connection weights almost immediately [see footnote 1]. The match between activation and connection weights caused by a successful transformation amplifies the representation, thereby projecting it to the third stage of the model [see footnote 2]. The transformation process is compatible with the depth transformations in Tarr’s handedness experiments (1995), as well as the linear deformations involved in the Lades et al. (1993) “lattice” recognition system; in addition, both processes fit nicely into Kosslyn et al.’s “pattern activation” subsystem in the ventral pathway.
The third system of the model consists of two parallel and lateralized subsystems. In the left hemisphere, a feature-based recognition system receives input from specific surface-combinations and will match them against a database of stored parts. By breaking apart these surface combinations into likely parts (on the basis of conversality or other contour-based principles emerging from Hebbian learning) the left-hemispheric feature processor will activate patterns that represent object parts; these candidate features are then projected to associative memory, which stores a comprehensive list of parts and relationships between them.
While the left hemisphere is engaged in part-based decomposition, the right hemisphere performs a more holistic analysis of the image by identifying the n principal components which could be combined to reproduce the normalized view of the image. In the case of face recognition, this process would involve combining the stored eigenvectors of the entire “face-space” in an attempt to match the current face to a certain accuracy criterion. As described in Calder & Young (2005), principle component analysis (PCA) has proven useful in machine vision applications, and FFA may perform an analogous computation. This implementation of holistic processing is also compatible with evidence that processing in the FFA is particularly sensitive to inversion (Gauthier, Curran, Curby, & Collins, 2003) possibly because efficient PCA requires that all images be normalized to a canonical view. Once a certain accuracy criterion is met, the principal components for a given image are then projected to associative memory.
This distinction between left- and right-hemispheric processes could be seen as controversial, given that some researchers believe FFA just happens to be the area of maximal activity for faces even though all visual representations are distributed, while others insist that the FFA is inherently selective for faces. Still others argue that we are all “face-experts” and that FFA is actually selective for expertise (Palmeri & Gauthier, 2004). However, an emerging body of ERP evidence suggests that FFA activity is actually selective for expertise, whether those experts are identifying Greebles, cars, dogs, cats, or faces (Tarr & Cheng, 2002, although see Xu, Liu, & Kanwisher 2005 for a different view). Further, this division is compatible with neuropsychological data in which dissociations in brain-damaged patients support two, but not one or three, distinct subsystems for object recognition (Farah, 1992; see Humphreys & Riddoch [in press] for possible exceptions, though these might instead be explained as semantic impairments in associative memory). Finally, evidence that viewpoint-dependent priming effects result only when items are presented to the right hemisphere (Burgund & Marsolek 2000) is consistent with the interpretation of hemispheric specialization given here.
The fourth and final step in this model is long-term associative memory. This region consists of feature units; once combinations of those features have become sufficiently activated, an object has been identified. After receiving projections from both left- and right-hemispheric recognition subsystems, this stage finds a constellation of features that both match those features identified in the left-hemispheric process and yet share historical correlations with the eigenvalues activated through the right-hemispheric process. In cases where feature information is ambiguous, associative memory may use holistic information to more strongly amplify one or another interpretation via its bidirectional connectivity with both components of the third stage.
Feature-based information may be sufficient for basic-level categorization, but subordinate-level distinctions may require holistic information to play a larger part. For certain domains, one of the two lateralized processes may be more heavily weighted by associative memory; in this way, domains in which more subordinate level distinctions are required (e.g., face recognition or other areas of expertise) will rely more heavily on right-hemispheric information. Although other “divisions of labor” between visual subsystems might also be capable of explaining the human data, this two-part architecture is both parsimonious and powerful.
In conclusion, this four-part model integrates neural, behavioral, ERP, and neuropsychological data on object recognition. Multiple view-dependent representations of surface-combinations are matched with incoming surface data through a transformation process. The results of this transformation process allow two parallel downstream units to bidirectionally excite associative memory, resulting in object recognition through constraint satisfaction between distinct subsystems. The model also suggests how both the multiple stored views of each object and the relative weighting of right- and left-hemispheric processes in recognizing objects from that domain may be altered with experience.
[Footnote 1] If no matching view is found, unstable activations will presumably oscillate for longer before settling into a lower energy state; this process may itself serve to modify weights enough to actually store a new view of the object.
[Footnote 2] While it may seem that the process of matching surface representations to a stored view would mean that object recognition already be completed, it is not necessarily so. At this point, visual data is nothing more than surface-combinations; characteristic or familiar views are simply arrangements of surfaces; neither of these is sufficient for object recognition. Bidirectional constraint satisfaction with stage 3 processes may also guide object transformation.
References:Biederman, I. (1995). Visual object recognition. In SF and DN Osherson (Eds.).An Invitation to Cognitive Science, 2nd edition, Volume 2., Cognition. MIT Press. Chapter 4, pp. 121-165.
Biederman, I., & Gerhardstein, P. C. (1993). Recognizing depth-rotated objects: Evidence and conditions for 3D viewpoint invariance. Journal of Experimental Psychology: Human Perception and Performance, 19, 1162-1182.
Burgund, E. D., & Marsolek, C. J. (2000). Viewpoint-invariant and viewpoint-dependent object recognition in dissociable neural subsystems. Psychonomic Bulletin & Review, 7, 480-489.
Calder AJ, Young AW. Understanding the recognition of facial identity and facial expression. Nat Rev Neurosci. 2005 Aug;6(8):641-51
Farah, MJ (1992). Is an object an object an object? Current Directionsin Psychological Science, 1:164-169.
Farah, MJ (2000). The Cognitive Neuroscience of Vision. Oxford: Blackwell Publishers.
Gauthier, I., Curran, T., Curby, K. M., & Collins, D. (2003). Perceptual interference supports a non-modular account of face processing. Nat Neurosci, 6(4), 428-432
Humphreys GW, & Riddoch MJ. Features, Objects, Action: The cognitive neuropsychology of visual object processing, 1984-2004. Cognitive Neuropsychology, 2006, 23 (0), 1–28
Kosslyn SM, Flynn RA, Amsterdam JB, Wang G. Components of high-level vision: a cognitive neuroscience analysis and accounts of neurological syndromes. Cognition. 1990 Mar;34(3):203-77
Lades, M., Vorbruggen, J.C., Buhmann, J., Lange, J., von der Malsburg, C., Wurtz, R.P., Konen, W., 1993. Distortion invariant object recognition in the dynamic link architecture. IEEE Transactions on Computers 42, 300–311.
Palmeri TJ, Gauthier I.(2004) Visual object understanding. Nat Rev Neurosci. 2004 Apr;5(4):291-303
Tarr, M. J. (1995). Rotating objects to recognize them: A case study of the role of viewpoint dependency in the recognition of three-dimensional objects. Psychonomic Bulletin and Review, 2, 55-82.
Tarr MJ, Cheng YD. Learning to see faces and objects. Trends Cogn Sci. 2003 Jan;7(1):23-30
Tarr, M. J., & Bülthoff, H. H. (1998). Image-based object recognition in man, monkey, and machine. Cognition, Special Issue on Image-based Object Recognition (Tarr & Bülthoff, eds.), 67 (1/2), 1-20.
Wolfe, J.M., Horowitz, T.S. (2004). What attributes guide the deployment of visual attention and how do they do it? Nature Reviews Neuroscience, 5 1-7.
Xu, Y., Liu, J., & Kanwisher, N. (2005). The M170 is Selective for faces, not for Expertise. Neuropsychologia, 43, 588-597
6 Comments:
I don't have time to give this a solid reading right now, but it certainly has one useful hallmark of a good theory: the citations list reads like a who's who of vision science!
Start with some classic Biederman, throw in a little Isabel Gauthier, some Glynn Humphreys, a dash of Kosslyn and Mike Tarr, and even some Jeremy Wolfe and Nancy Kanwisher for good measure! :-)
Slightly more seriously, I think the fact that Nancy Kanwisher was invited by Science to write the review of the recent Tsao article showing face sensitivity in monkey brains shows the the field is tilting slightly more toward viewing the FFA as a predominantly face area, rather than an area of expertise. After all, they could have invited Palmeri or Gauthier to write the review, as they would usually have done. Personally I'm sympathetic to the expertise account of the FFA, but who knows.
I too noticed Kanwisher's review in Science, and thought it strange. I think she kind of avoided the real issue (whether FFA is specialized for faces or specialized for expertise) in her editorial though. I mean, does anyone think it's really not specialized at all? That seemed to be the straw man against whom she was arguing....
I don't know about the later stages, but I think it is likely that detection of contours/gradients occurs at an early stage, maybe even prior to V1.
Yep I meant to say that the first step of object recognition in inferotemporal cortex is the extraction of surface information from contours. We know that contour information does not occur within IT, because IT lesioned monkeys are still able to do basic obj. recognition on the basis of contour.
Bob - just found your page and your new MiniMind project. Fascinating stuff - but i don't understand how I can query it myself, and there doesn't seem to be an executable. What am i missing?
Ok, I'll put up an installable version of MiniMind so that people can play around with it.
MiniMind doesn't do anything highly intelligent, but it is a useable semantic network which expands its corpus automatically. I've had it running for a few days now, and its generated almost 10,000 new mindpixels from Wikipedia.
Post a Comment
<< Home